The Intersection of Medical Imaging and AI
Medical imaging and AI are transforming wound care by offering clinicians advanced tools for assessing and treating complex injuries with greater precision. AI’s ability to automate image analysis and enhance diagnostic imaging has improved wound assessment, detection of complications, and personalized treatment planning. As AI technology continues to evolve, ongoing research and development focus on refining these tools, integrating real-time feedback, and addressing ethical challenges like data privacy and transparency. This exploration of AI’s expanding role highlights its potential to further revolutionize wound care.
Evolution of Imaging Techniques
The evolution of imaging techniques has dramatically transformed wound care, particularly for burn clinicians and ER doctors who treat complex injuries. Starting with X-rays, early imaging provided basic insight into skeletal injuries but lacked the ability to visualize soft tissue damage accurately. As technology advanced, imaging modalities evolved to offer more detailed, real-time information about tissue integrity, blood flow, and infection risks, critical for wound management.
Key advancements in imaging include:
- X-rays: Initially used to identify fractures, X-rays offered limited value for wound assessment due to their focus on bones rather than soft tissue. While useful for determining underlying skeletal damage, X-rays could not capture the intricacies of wounds or burns.
- Ultrasound imaging: The introduction of ultrasound offered non-invasive visualization of soft tissue, blood vessels, and wound depth. This technology allowed clinicians to evaluate wound severity without surgical intervention, making it especially valuable in burn care.
- CT and MRI scans: Computed tomography (CT) and magnetic resonance imaging (MRI) further revolutionized wound imaging by offering detailed cross-sectional views of both hard and soft tissues. These modalities enabled better evaluation of deep tissue damage, infection, and necrosis in complex wounds.
- Digital imaging and 3D wound mapping: Modern wound care heavily relies on digital imaging, including 3D wound mapping and infrared thermal imaging. These tools provide high-resolution views of the wound surface and internal structures, allowing clinicians to assess healing progress, detect early signs of infection, and plan surgical interventions.
The impact of digital imaging cannot be overstated. It has streamlined wound assessments, improved diagnostic accuracy, and enabled more precise treatment plans. As imaging technology continues to advance, clinicians will have even greater tools to enhance wound care and patient outcomes.
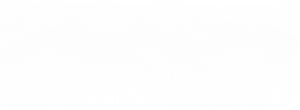
More about Deepview
Learn more about our DeepView® technology
Request a Demo
Looking to learn more about DeepView® technology, or eager to see it in action?
AI’s Role in Modern Imaging
Artificial intelligence is rapidly changing the landscape of modern wound imaging, providing clinicians with advanced tools for more accurate and efficient wound assessment. In burn care and emergency settings, AI-driven technologies are streamlining the imaging process by automating analysis and enhancing the clarity of captured images. This automation allows clinicians to interpret wounds with greater precision, leading to faster, more informed decisions and improved patient outcomes.
Key benefits of AI in imaging include:
- Automated image analysis: AI algorithms can process and analyze wound images faster and more consistently than manual methods. These systems measure wound dimensions, assess tissue viability, and even detect subtle changes in healing over time. Automated analysis reduces human error, ensures standardized evaluations, and allows clinicians to focus on making informed treatment decisions.
- Enhanced image quality: AI-powered imaging systems use advanced algorithms to enhance image clarity and resolution. This ensures that clinicians get the most detailed view possible, even in challenging conditions like low light or during patient movement. This is especially helpful in difficult-to-capture situations, such as wounds with irregular surfaces or deep burns, giving clinicians a clearer view for diagnosis and treatment planning.
- Improved interpretation: By leveraging AI, clinicians can interpret images with the support of predictive analytics. AI tools can compare current wound images with vast databases of historical data, identifying patterns and suggesting potential treatment plans based on similar cases. This data-driven approach supports clinicians in making evidence-based decisions, ultimately leading to personalized and effective care.
As AI continues to evolve, it is expected to play an even more critical role in wound imaging. Its ability to automate analysis, enhance image quality, and provide data-driven insights makes it a powerful asset in modern wound management, helping clinicians address complex cases with greater confidence and accuracy.
Machine Learning and Its Applications in Medical Imaging
Machine learning is revolutionizing medical imaging, providing doctors with tools to assess wounds and injuries more accurately and efficiently. By using deep learning algorithms, these systems can analyze vast amounts of imaging data to detect patterns, classify wounds, and assist in diagnosis with minimal human intervention.
Deep learning algorithms, a subset of machine learning, are trained on extensive datasets of medical images. For wound care, these models learn to identify tissue damage, infection risks, and healing potential, enabling automated image analysis. This significantly reduces time spent on manual assessments, allowing clinicians to focus on treatment decisions.
Research and development in this field continue to drive innovation. AI-powered imaging tools are advancing rapidly, providing improved accuracy and speed in identifying wound characteristics. Continuous learning models refine their capabilities as new data emerges, making them more adaptable to various wound types and patient conditions.
Deep Learning Algorithms
Deep learning algorithms offer more accurate, efficient, and automated assessments. In wound care, burn treatment, and emergency settings, these advancements are helping clinicians evaluate injuries more effectively. Deep learning algorithms, a subset of machine learning, process large volumes of medical imaging data to identify patterns, detect anomalies, and assist in diagnosis, often with minimal human intervention.
Training AI models with medical data is essential to their success:
- Data-driven training: Deep learning algorithms require vast amounts of annotated medical images to learn and improve their performance. In wound care, these models are trained on thousands of images, including burns, lacerations, and ulcers, to recognize key features such as depth, infection risk, and healing potential. This allows for automated wound assessment that supports clinical decision-making.
- Continuous learning: As new data becomes available, these algorithms continuously refine their accuracy. The more diverse the data, the better the AI models become at generalizing to various wound types, skin conditions, and patient demographics, reducing the potential for diagnostic errors.
Machine learning has already seen successful implementations in radiology:
- Enhanced radiology applications: AI models in radiology are currently used to detect fractures, tumors, and other abnormalities on X-rays, CT scans, and MRIs. Their high accuracy and speed in identifying subtle patterns make them indispensable in busy clinical environments. Similar applications are being developed for wound imaging, where AI models assist in assessing tissue damage and predicting healing outcomes.
By leveraging deep learning in medical imaging, clinicians can benefit from faster, more precise diagnoses and improved patient outcomes. The ongoing development of these AI-driven tools promises to revolutionize wound care by offering reliable, data-backed insights into injury management.
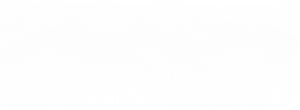
More about Deepview
Learn more about our DeepView® technology
Request a Demo
Looking to learn more about DeepView® technology, or eager to see it in action?
Research and Development
Research and development in medical imaging and artificial intelligence are advancing rapidly, offering new opportunities for burn clinicians and ER doctors to improve wound assessment and treatment. Ongoing projects are focused on refining deep learning algorithms to enhance diagnostic imaging and automate complex wound data analysis more efficiently. These AI-powered medical tools are trained on vast biomedical imaging datasets, learning to detect patterns such as tissue damage, infection, and healing progress.
One key area of AI-enabled medical research is improving the detection of subtle wound characteristics that are challenging to identify with traditional methods. For instance, advanced algorithms are being developed to assess tissue perfusion and predict complications like infection or delayed healing. These innovations in imaging analysis give clinicians a more comprehensive understanding of wound health, leading to better treatment decisions.
Looking ahead, AI’s role in medical imaging will expand further. Future developments may integrate real-time imaging feedback with wearable devices, allowing continuous wound monitoring and early detection of complications. As AI-enabled medical research continues, these applications will offer faster, more personalized care, improving outcomes in health management for complex wounds across various clinical settings.
Challenges and Future Directions
Ethical and Regulatory Considerations
As AI continues to revolutionize medical imaging, particularly in wound care, it brings new challenges and future directions that must be addressed. Ethical and regulatory considerations are at the forefront of AI’s integration into healthcare. Burn clinicians and ER doctors who rely on AI-powered medical imaging tools must navigate issues such as data privacy and the transparency of AI systems.
Data privacy concerns are critical when using AI-enabled medical tools. These systems require vast amounts of sensitive patient data to train algorithms, making the protection of this information a top priority. Ensuring that data is securely stored, anonymized, and compliant with health regulations like HIPAA is essential for maintaining patient trust.
Another key challenge is ensuring AI transparency and accountability. Clinicians need to understand how AI algorithms arrive at their conclusions to trust the results. The “black box” nature of many AI models can make it difficult to explain their decisions, which raises concerns about potential biases or errors. AI systems must provide clear, interpretable insights to allow clinicians to verify the validity of their recommendations and adjust treatments accordingly.
The integration of medical imaging and AI has profoundly transformed wound care, enabling more precise, efficient, and personalized treatment approaches. From the evolution of imaging techniques like X-rays to advanced AI-driven tools, clinicians now have access to enhanced diagnostic capabilities. AI’s role in automating image analysis, improving image quality, and offering predictive insights has streamlined clinical workflows, improving patient outcomes. However, as AI continues to evolve, addressing ethical and regulatory concerns such as data privacy and algorithmic transparency is essential to ensure safe, reliable, and accountable applications. By focusing on these areas, the future of AI in medical imaging looks promising, offering even greater potential for healthcare advancements.