Artificial intelligence in medical diagnosis is revolutionizing the field of healthcare, offering new levels of accuracy and efficiency. AI technologies, particularly in medical diagnostics, are transforming how diseases are detected, analyzed, and treated. By leveraging machine learning and deep learning algorithms, AI can process vast amounts of data swiftly and accurately, providing healthcare providers with invaluable insights. These advancements are not only enhancing the precision of diagnoses but also enabling early detection and personalized treatment plans. This article explores the various ways AI is reshaping medical diagnostics, with a focus on its applications, benefits, and the challenges that come with integrating these cutting-edge technologies into clinical practice.
How AI is Revolutionizing Medical Diagnostics
The evolution of AI in healthcare has been transformative, especially in the field of medical diagnostics. Initially, AI was primarily used for administrative tasks, but its role has expanded significantly. Now, AI and machine learning algorithms analyze vast amounts of data quickly and accurately, assisting healthcare providers in making more informed decisions. These technologies can process medical images, recognize patterns, and even predict disease outcomes, revolutionizing the practice of medicine.
Role of AI in Diagnosis:
- Enhanced Accuracy: AI algorithms improve diagnostic accuracy by analyzing complex medical data, reducing human error.
- Early Detection: Machine learning models can identify early signs of diseases such as cancer or heart disease, allowing for timely intervention.
- Personalized Medicine: AI tailors treatments based on individual patient data, leading to more effective and personalized care plans.
- Efficiency: Automated systems speed up the diagnostic process, freeing up healthcare providers to focus on patient care.
Benefits of AI in Healthcare:
- Data Analysis: AI processes large datasets from electronic health records (EHRs), providing insights that are difficult to achieve manually.
- Imaging: Advanced AI tools enhance the interpretation of medical images, aiding radiologists in identifying abnormalities.
- Predictive Analytics: Predictive models forecast disease progression, helping in preventive care and better resource allocation.
- Clinical Decision Support: AI systems provide evidence-based recommendations, supporting clinicians in making more informed decisions.
Challenges:
- Integration: Integrating AI systems with existing healthcare infrastructure requires significant investment and training.
- Data Privacy: Ensuring the security of sensitive patient data is paramount.
- Regulation: Developing appropriate regulatory frameworks to oversee AI applications in medicine is essential.
In conclusion, AI is revolutionizing medical diagnostics by enhancing accuracy, enabling early detection, and personalizing patient care. Healthcare providers must embrace these advancements, addressing challenges such as integration, data privacy, and regulation, to fully realize the potential of AI in transforming health care.
Artificial Intelligence is Enhancing Diagnostic Accuracy
Artificial intelligence (AI) is significantly enhancing diagnostic accuracy in the medical field, often outperforming traditional methods. For instance, in radiology, AI-powered algorithms can analyze medical images with remarkable precision. Studies have shown that AI systems detect breast cancer in mammograms more accurately than human radiologists. These AI tools analyze thousands of images to recognize patterns and subtle changes that might be overlooked by the human eye.
AI often outperforms the accuracy of traditional methods in burn and wound management. AI-powered tools use advanced machine learning algorithms to analyze images of wounds, providing rapid and precise assessments. These tools can identify infection, determine the depth of burns, and monitor healing progress with greater accuracy than conventional techniques.
Moreover, AI algorithms have been developed to predict wound healing outcomes. These models use patient data, including demographics, wound characteristics, and comorbidities, to forecast healing trajectories. Such predictions help clinicians tailor treatment plans, optimize resource allocation, and improve patient outcomes.
AI also excels in detecting diabetic foot ulcers. Machine learning models trained on large datasets of foot images can accurately classify ulcer severity and recommend appropriate debridement techniques. This level of precision reduces the risk of complications, such as infections and amputations, thereby enhancing patient care.
In burn management, AI enhances diagnostic accuracy by analyzing burn images to determine the need for surgical intervention. Traditional methods rely on subjective visual assessments, which can vary between clinicians. AI tools, however, provide consistent and objective evaluations, improving the accuracy of burn depth assessment and surgical planning.
Furthermore, AI facilitates early detection of infections in chronic wounds. Machine learning algorithms analyze wound exudate and other clinical data to identify signs of infection before they become clinically apparent. Early detection allows for prompt treatment, reducing the risk of severe complications and promoting faster recovery.
By leveraging machine learning and advanced data analysis, AI tools provide healthcare providers with precise, timely, and actionable insights. Integrating AI into clinical practice enhances the quality of medical care, ultimately improving health outcomes for patients with burns and wounds. Spectral Ai’s patented DeepView® technology is an AI wound diagnostics platform that combines AI algorithms and medical imaging for diagnostic wound healing predictions.
DeepView®
A new approach to diagnostic wound assessment
Our patented DeepView® technology is an AI wound diagnostics platform that combines AI algorithms and medical imaging for diagnostic wound healing predictions.
Speeding Up Disease Diagnosis
AI is revolutionizing disease diagnosis in burn and wound management, significantly speeding up the process and enhancing accuracy. AI-powered tools, leveraging advanced machine learning algorithms, can analyze wound images swiftly, providing precise assessments. These tools identify infection, evaluate burn depth, and monitor healing progress much faster than traditional methods.
Examples of AI in Practice:
- Telemedicine: AI applications enable remote diagnosis and management of wounds. High-resolution images analyzed by AI systems can detect subtle changes indicating complications, allowing timely interventions, especially in remote areas with limited access to specialized care.
- Predictive Modeling: AI algorithms predict wound healing outcomes by analyzing patient data, including demographics, wound characteristics, and comorbidities. These predictions help clinicians tailor treatment plans, optimize resource use, and improve patient outcomes.
- Diabetic Foot Ulcers: AI accurately classifies ulcer severity and recommends appropriate debridement techniques by analyzing large datasets of foot images. This precision reduces the risk of infections and amputations, enhancing patient care.
In burn management, AI tools determine the need for surgical intervention by analyzing burn images. Traditional visual assessments can be subjective and vary between clinicians. AI provides consistent, objective evaluations, improving burn depth assessment and surgical planning accuracy. This accelerates the diagnostic process, ensuring timely and appropriate treatment.
Furthermore, AI aids in early infection detection in chronic wounds. Machine learning algorithms analyze wound exudate and clinical data to identify signs of infection before they become apparent. Early detection allows for prompt treatment, reducing severe complications and promoting faster recovery.
AI in Disease Prediction and Prevention
AI offers unprecedented advancements in medical care by transforming disease prediction and prevention. By leveraging machine learning algorithms, AI analyzes vast datasets to identify patterns and predict potential health issues before they manifest, enabling proactive interventions.
Predictive Analytics in Wound Management:
- Infection Risk Prediction: AI models can assess patient data and wound characteristics to predict infection risks. Early detection allows clinicians to implement preventive measures, reducing the incidence of severe infections.
- Healing Trajectory Forecasts: Machine learning algorithms evaluate various factors, such as patient demographics, wound type, and treatment history, to predict healing outcomes. This enables healthcare providers to customize care plans, optimizing recovery times and improving health outcomes.
- Burn Complication Prevention: AI tools analyze burn injury data to predict complications, such as hypertrophic scarring or contractures. Early identification of at-risk patients allows for timely interventions, enhancing long-term results.
Applications in Disease Prevention:
- Personalized Treatment Plans: AI-driven insights help clinicians develop personalized care strategies by considering individual patient profiles and potential risk factors. This targeted approach ensures efficient resource utilization and improved patient satisfaction.
- Proactive Health Monitoring: Continuous monitoring of patient health data through AI-powered systems enables early detection of deviations from normal healing patterns. Prompt alerts allow for immediate corrective actions, preventing complications and promoting faster recovery.
- Telemedicine Integration: AI enhances telemedicine platforms by providing real-time analysis of wound images and patient data. Remote consultations supported by AI diagnostics ensure timely and accurate care, particularly for patients in underserved regions.
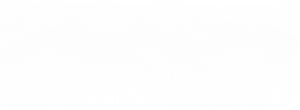
More about Deepview
Learn more about our DeepView® technology
Request a Demo
Looking to learn more about DeepView® technology, or eager to see it in action?
AI Technologies Powering Diagnostic Innovations
AI technologies are driving diagnostic innovations in burn and wound management, enhancing accuracy and efficiency in medical care. Advanced machine learning algorithms analyze extensive datasets, identifying patterns and insights that significantly improve diagnosis and treatment plans.
Key AI Technologies in Diagnostics:
- Image Analysis: AI-powered tools analyze medical images with exceptional precision. For instance, in wound management, AI can assess wound size, depth, and healing progression from photographs, providing accurate and consistent evaluations that aid in treatment planning.
- Predictive Analytics: Machine learning models predict disease progression and patient outcomes by analyzing historical and real-time data. These predictions help clinicians anticipate complications and adjust care plans proactively, optimizing patient health outcomes.
- Natural Language Processing (NLP): NLP algorithms process and analyze clinical notes and electronic health records (EHRs), extracting valuable information that supports diagnostic decisions. This technology enables a comprehensive understanding of patient history and current health status, facilitating accurate diagnoses.
- Telemedicine Integration: AI enhances telemedicine platforms by offering real-time analysis of wound images and patient data during remote consultations. This integration ensures timely and accurate assessments, especially beneficial for patients in remote or underserved areas.
Applications in Burn and Wound Management:
- Infection Detection: AI models identify early signs of infection by analyzing wound images and clinical data, allowing for prompt intervention and reducing the risk of severe complications.
- Healing Rate Assessment: AI algorithms evaluate healing rates by comparing current and past wound images, providing insights into treatment effectiveness and necessary adjustments.
- Risk Stratification: AI systems stratify patients based on risk factors, enabling personalized care plans that address individual needs and improve overall treatment outcomes.
Machine Learning and Deep Learning to Diagnose Disease
Machine learning and deep learning offer unprecedented accuracy and speed in medical care. These AI-driven technologies analyze vast amounts of data to identify patterns and predict outcomes, significantly enhancing diagnostic capabilities in burn and wound management.
Machine learning algorithms excel at processing large datasets, including patient histories, wound images, and treatment responses. By identifying subtle correlations within this data, these models can predict wound healing trajectories, assess infection risks, and recommend personalized treatment plans. For example, machine learning models can analyze photographic images of wounds, determining the depth and severity with greater accuracy than traditional methods.
Deep learning, a subset of machine learning, utilizes neural networks to mimic the human brain’s processing. This technology is particularly effective in image recognition tasks. In burn and wound management, deep learning algorithms can scrutinize high-resolution images to detect minute changes in wound appearance, signaling infection or other complications. These insights enable healthcare providers to intervene promptly, improving patient outcomes.
Moreover, deep learning systems can continuously learn and improve from new data, becoming more accurate over time. As these models are exposed to more patient data, their diagnostic precision increases, leading to better health outcomes. Integrating machine learning and deep learning into clinical practice empowers healthcare providers to deliver high-quality, personalized care.
Natural Language Processing (NLP) in Health Care
Natural Language Processing (NLP) enables more efficient and accurate analysis of medical information. For healthcare providers specializing in burn and wound management, NLP offers powerful tools to enhance patient care and streamline administrative tasks. This AI technology processes and interprets vast amounts of unstructured text data from electronic health records (EHRs), clinical notes, and research articles, extracting valuable insights that support clinical decision-making.
NLP algorithms can identify relevant patient information from EHRs, such as wound characteristics, treatment history, and comorbidities, to create comprehensive patient profiles. These profiles help clinicians tailor treatment plans more precisely, improving patient outcomes. For instance, NLP can quickly summarize a patient’s medical history, highlighting previous wound treatments and responses, which is crucial for developing effective care strategies.
Furthermore, NLP enhances the efficiency of documentation by automating the extraction of key data points from clinical notes. This automation reduces the time clinicians spend on administrative tasks, allowing them to focus more on patient care. NLP tools can also identify patterns and trends in large datasets, providing insights into wound healing rates and identifying potential complications early.
In addition, NLP facilitates better communication and collaboration among healthcare teams. By accurately translating and summarizing complex medical information, NLP ensures that all team members have access to consistent and up-to-date patient data. This capability is particularly beneficial in multidisciplinary settings where coordinated care is essential for managing complex wounds.
NLP also supports research by enabling the analysis of vast amounts of scientific literature. Researchers can quickly identify relevant studies, extract critical information, and stay informed about the latest advancements in burn and wound management.
By leveraging advanced machine learning and deep learning algorithms, AI technologies can analyze vast datasets, recognize complex patterns, and predict disease outcomes with remarkable precision. These capabilities are transforming healthcare, particularly in burn and wound management, where AI facilitates early detection, personalized treatment plans, and improved patient outcomes. Despite the challenges of integrating AI into existing medical infrastructures, including issues of data privacy and the need for robust regulatory frameworks, the benefits are clear. AI-driven diagnostic innovations are not only improving the quality of care but also enabling healthcare providers to make more informed decisions swiftly. As AI continues to evolve, its role in medical diagnostics will undoubtedly expand, paving the way for even more significant advancements in medicine and overall health care. Healthcare providers must embrace these technologies to stay at the forefront of medical innovation and deliver superior patient care.
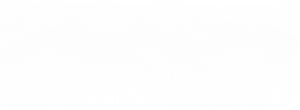
More about Deepview
Learn more about our DeepView® technology
Request a Demo
Looking to learn more about DeepView® technology, or eager to see it in action?